좋은 spark 2.0 소개 자료가 있다.
성능이 월등히 좋아졌다.
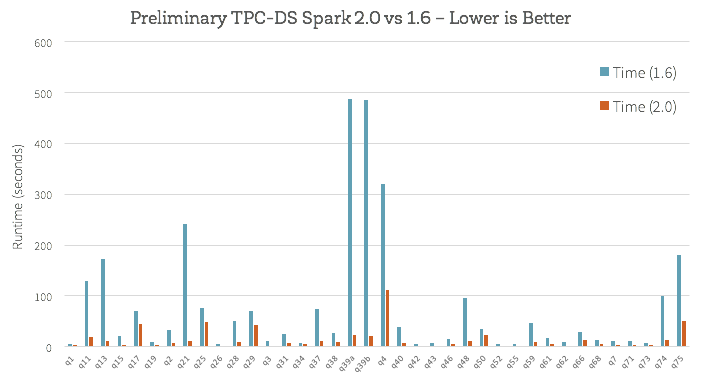
primitive | Spark 1.6 | Spark 2.0 |
---|---|---|
filter | 15ns | 1.1ns |
sum w/o group | 14ns | 0.9ns |
sum w/ group | 79ns | 10.7ns |
hash join | 115ns | 4.0ns |
sort (8-bit entropy) | 620ns | 5.3ns |
sort (64-bit entropy) | 620ns | 40ns |
sort-merge join | 750ns | 700ns |
API가 좋아졌다.
DataFrame이 쓰기 편해졌고, HiveContext(SQLContext) 대신 SparkSession이 새로 추가되었다.
- Unifying DataFrames and Datasets in Scala/Java: Starting in Spark 2.0, DataFrame is just a type alias for Dataset of Row. Both the typed methods (e.g.
map
,filter
,groupByKey
) and the untyped methods (e.g.select
,groupBy
) are available on the Dataset class. Also, this new combined Dataset interface is the abstraction used for Structured Streaming. Since compile-time type-safety in Python and R is not a language feature, the concept of Dataset does not apply to these languages’ APIs. Instead, DataFrame remains the primary programing abstraction, which is analogous to the single-node data frame notion in these languages. Get a peek from a Dataset API notebook. - SparkSession: a new entry point that replaces the old SQLContext and HiveContext. For users of the DataFrame API, a common source of confusion for Spark is which “context” to use. Now you can use SparkSession, which subsumes both, as a single entry point, as demonstrated in this notebook. Note that the old SQLContext and HiveContext are still kept for backward compatibility.
- Simpler, more performant Accumulator API: We have designed a new Accumulator API that has a simpler type hierarchy and support specialization for primitive types. The old Accumulator API has been deprecated but retained for backward compatibility
- DataFrame-based Machine Learning API emerges as the primary ML API: With Spark 2.0, the spark.ml package, with its “pipeline” APIs, will emerge as the primary machine learning API. While the original spark.mllib package is preserved, future development will focus on the DataFrame-based API.
- Machine learning pipeline persistence: Users can now save and load machine learning pipelines and models across all programming languages supported by Spark.
- Distributed algorithms in R: Added support for Generalized Linear Models (GLM), Naive Bayes, Survival Regression, and K-Means in R.
'scala' 카테고리의 다른 글
[scala] scala.collection.GenTraversableOnce[?] 해결 하기 (0) | 2017.03.07 |
---|---|
[scala] List와 Array의 lift 메소드 (0) | 2017.03.04 |
[spark] spark의 OutOfMemoryError 발생시 (0) | 2017.02.24 |
[spark] spark summit 자료 (0) | 2017.02.22 |
[scala] Array.transpose 예시 (0) | 2017.02.17 |